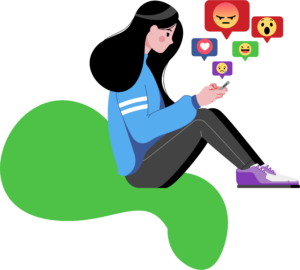
In the digital age, where text-based communication dominates, understanding the underlying emotions in written content has never been more crucial. Whether it’s social media posts, online articles, or personal blogs, the emotional tone of text can significantly influence the reader’s perception and engagement. This is where the Linguistic Inquiry and Word Count (LIWC) comes into play, a powerful tool for extracting and analyzing emotions in text.
What is LIWC?
LIWC, developed by James W. Pennebaker and colleagues, is a text analysis software that categorizes words into various psychological dimensions, including emotions. It operates on a simple yet profound premise: the language we use reflects our thoughts, feelings, and personalities. LIWC includes two sentiment categories (positive, negative) and three negative emotion categories (anger, anxiety, sad) [1].
How Does LIWC Extract Emotions?
LIWC contains a specialized dictionary with thousands of words categorized into different emotion categories. When analyzing a text, LIWC counts the frequency of words that fall into these categories, offering a quantitative measure of the emotional content. For instance, words like “happy,” “joy,” and “excited” would contribute to a higher score in positive emotions, while words such as “angry,” “hate,” and “upset” would indicate negative emotions.
For example, the below screenshot shows an Instagram post by the New York Times (@nytimes). Given the body text of this news post (194 words), LIWC returns the following values: 1.03% (2 words) for positive sentiment, 3.61% (7 words) for negative sentiment, 0% for anxiety, 1.55% (3 words) for anger, 0% for sadness [1].
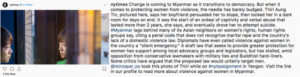
Applications in Various Fields
The applications of LIWC are diverse and impactful. In psychology, it helps in understanding emotional expressions in therapeutic writing. Marketers use it to gauge customer sentiment in product reviews. In journalism, it can analyze the emotional tone of news articles, shaping how stories are communicated. Even in personal relationships, LIWC can provide insights into the emotional health of communication.
Case Study: Social Media and News [1]
A study analyzed the emotional content of news posts across different social media platforms using LIWC. By examining posts and comments from major news outlets, the study revealed interesting patterns in how emotions are expressed and perceived on platforms like Facebook, Twitter, and Instagram. For instance, Instagram posts showed a prevalence of positive emotions, while Twitter presented a more balanced emotional expression.
Implications for Content Creators
Understanding the emotional content of text can be a game-changer for content creators. It allows them to tailor their message to resonate with their audience emotionally. For news organizations, this means crafting stories that engage readers on an emotional level, potentially increasing readership and shareability. For example, news outlets tend to have a specific style, with outlets such as @usatoday using the largest number of positive lexicons, and @nytimes and @chicagotribune tending to use the smallest number of emotional words [1].
The Future of Textual Emotional Analysis
As technological progress continues, advanced tools such as LIWC evolve alongside, gaining sophistication and providing more profound insights into the intricate landscape of emotions conveyed in text. The incorporation of large language models (LLMs), a cutting-edge facet of machine learning and natural language processing, holds the potential to significantly elevate the precision and breadth of emotional analysis. These models, trained on vast datasets, are adept at understanding and interpreting the nuances of human language, thus offering a more dynamic and contextually aware approach to emotion detection and analysis in text.
Conclusion
LIWC opens a window into the emotional landscape of text, offering valuable insights across various domains. By understanding the emotional content of written communication, we can foster better engagement, empathy, and understanding in our increasingly digital world.
[1] Aldous, K. K., An, J., & Jansen, B. J. (2022). Measuring 9 emotions of news posts from 8 news organizations across 4 social media platforms for 8 months. ACM Transactions on Social Computing (TSC), 4(4), 1-31.